Our Health In Our Hands
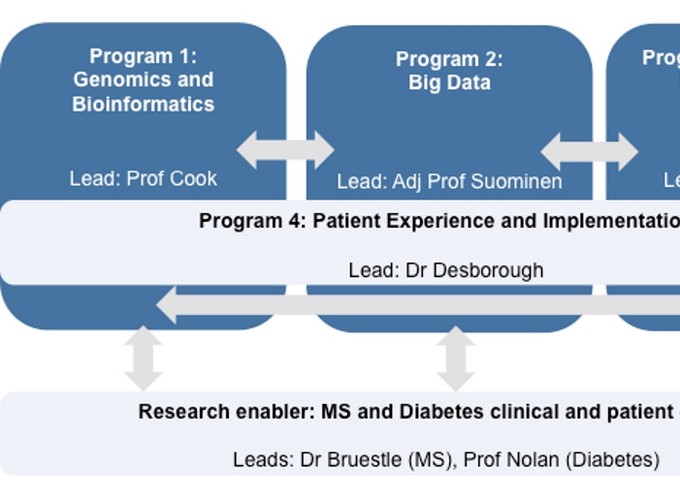
Our Health In Our Hands
Publications
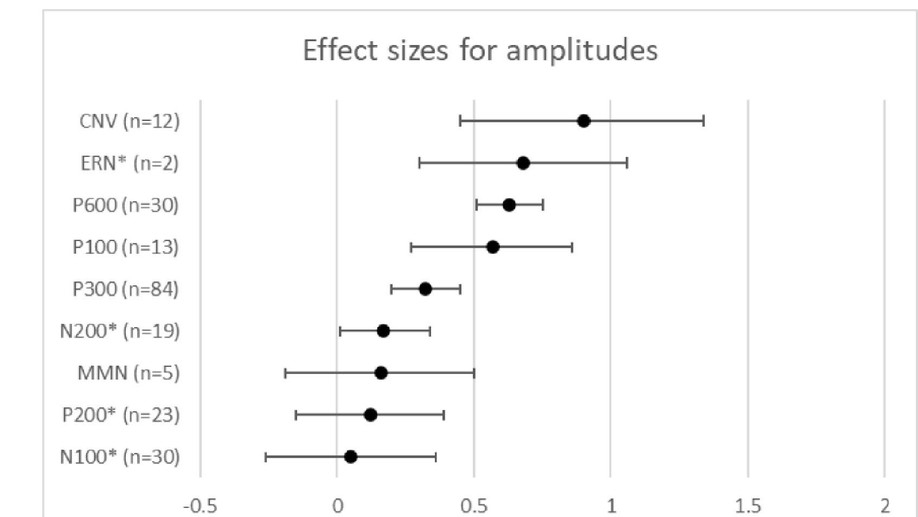
The use of event-related potentials in the investigation of cognitive performance in people with Multiple Sclerosis: Systematic review
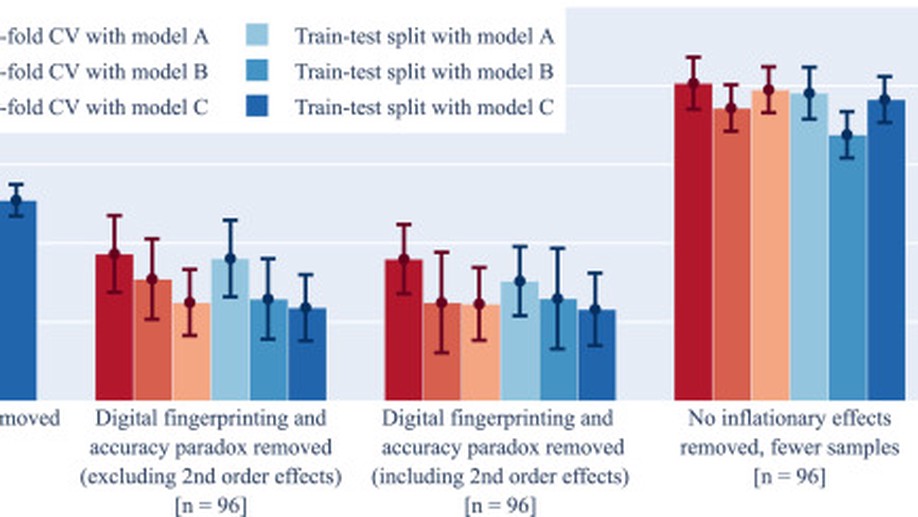
Has machine learning over-promised in healthcare?: A critical analysis and a proposal for improved evaluation, with evidence from Parkinson’s disease
Evaluating Effects of Resting-State Electroencephalography Data Pre-Processing on a Machine Learning Task for Parkinson’s Disease
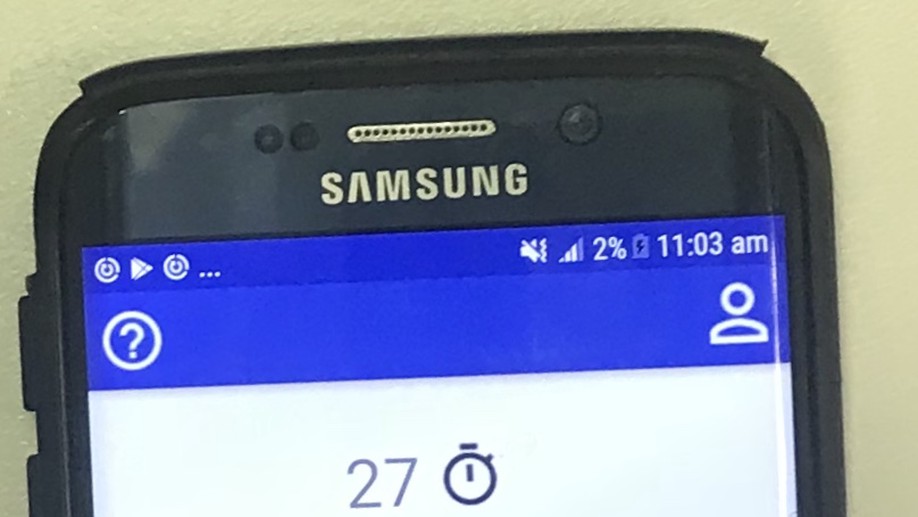
Finger tapping measures for Parkinson’s disease: Preliminary evaluation of an Android application for data collection in Australia
The use of event-related potentials and machine learning to improve diagnostic testing and prediction of disease progression in Parkinson's disease
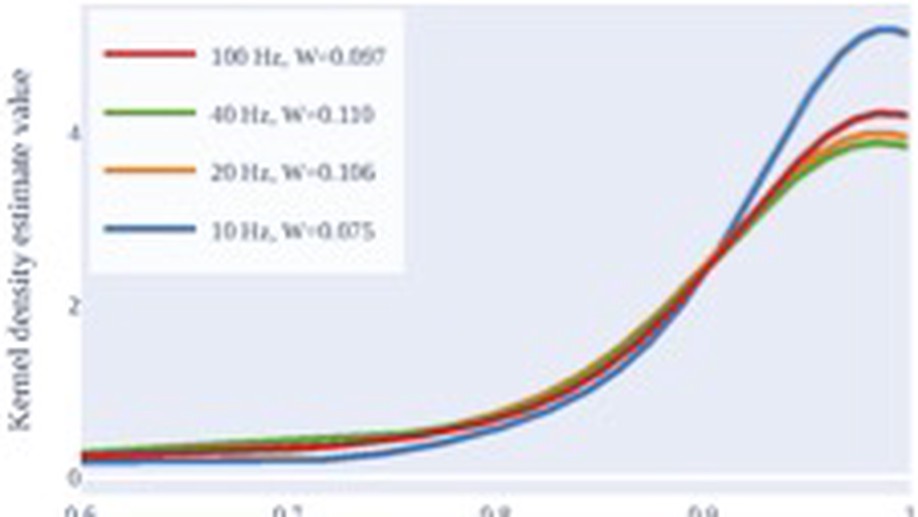
Which features of postural sway are effective in distinguishing Parkinson's disease from controls? An experimental investigation
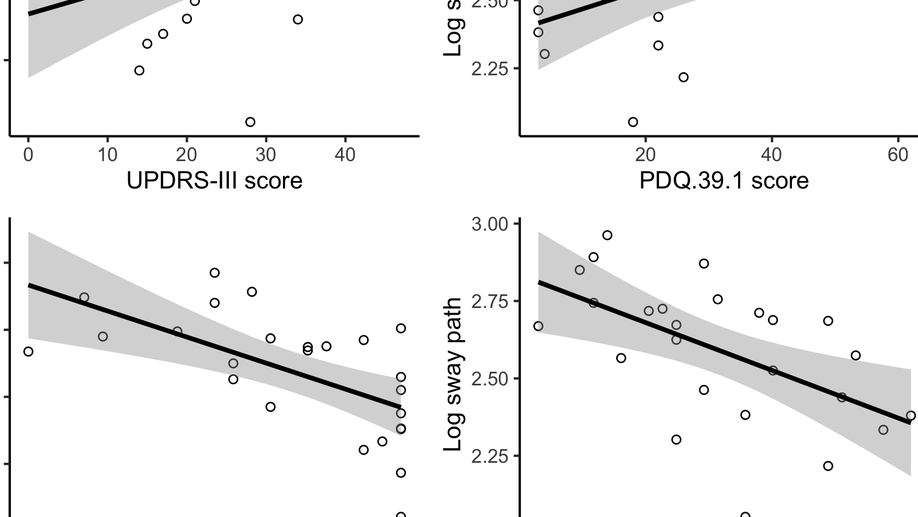
Postural sway correlates with cognition and quality of life in Parkinson’s disease
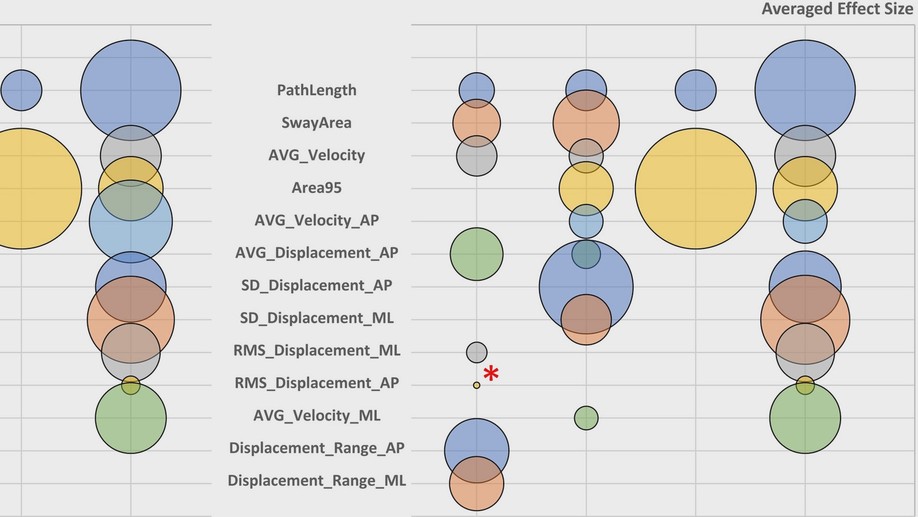
Which features of postural sway are effective in distinguishing Parkinson's disease from controls? A systematic review
Background: Postural sway may be useful as an objective measure of Parkinson’s disease (PD). Existing studies have analyzed many different features of sway using different experimental paradigms. We aimed to determine what features have been used to measure sway and then to assess which feature(s) best differentiate PD patients from controls. We also aimed to determine whether any refinements might improve discriminative power and so assist in standardizing experimental conditions and analysis of data.
Methods: In this systematic review of the literature, effect size (ES) was calculated for every feature reported by each article and then collapsed across articles where appropriate. The influence of clinical medication status, visual state, and sampling rate on ES was also assessed. Results: Four hundred and forty‐three papers were retrieved. 25 contained enough information for further analysis. The most commonly used features were not the most effective (e.g., PathLength, used 14 times, had ES of 0.47, while TotalEnergy, used only once, had ES of 1.78). Increased sampling rate was associated with increased ES (PathLength ES increased to 1.12 at 100 Hz from 0.40 at 10 Hz). Measurement during “OFF” clinical status was associated with increased ES (PathLength ES was 0.83 OFF compared to 0.21 ON). Conclusions: This review identified promising features for analysis of postural sway in PD, recommending a sampling rate of 100 Hz and studying patients when OFF to maximize ES. ES complements statistical significance as it is clinically relevant and is easily compared across experiments. We suggest that machine learning is a promising tool for the future analysis of postural sway in PD.